https://blog.finxter.com/wp-content/plugins/wp-youtube-lyte/lyteCache.php?origThumbUrl=https%3A%2F%2Fi.ytimg.com%2Fvi%2F-JKVy_HliQE%2F0.jpg
When working with data in Python, one of the most powerful tools at your disposal is the pandas DataFrame.
A DataFrame is a two-dimensional data structure, which essentially looks like a table with rows and columns. This versatile data structure is widely used in data science, machine learning, and scientific computing, among other data-intensive fields. It combines the best features of SQL tables and spreadsheets, offering a more efficient way to work with large volumes of structured data.
I recorded my short 5-minute Pandas introduction video a while ago but it’s still relevant today:

As you dive deeper into pandas, you’ll find that DataFrames give you flexibility and high performance, allowing you to easily perform various operations, such as filtering, sorting, and aggregating data.
DataFrames also support a wide range of data types, from numerical values to timestamps and strings, making it suitable for handling diverse data sources.
You can easily create a DataFrame object using the pd.DataFrame()
function, providing it with data in several formats, such as dictionaries, NumPy arrays, or even CSV files.
In this article, you can familiarize yourself with their key components and features. Understanding how to index, slice, and concatenate DataFrames will enable you to manipulate data efficiently and optimize your workflow.
Moreover, pandas offers a wealth of built-in functions and methods, empowering you to perform complex data analysis and visualization tasks.
Pandas DataFrame Object Fundamentals
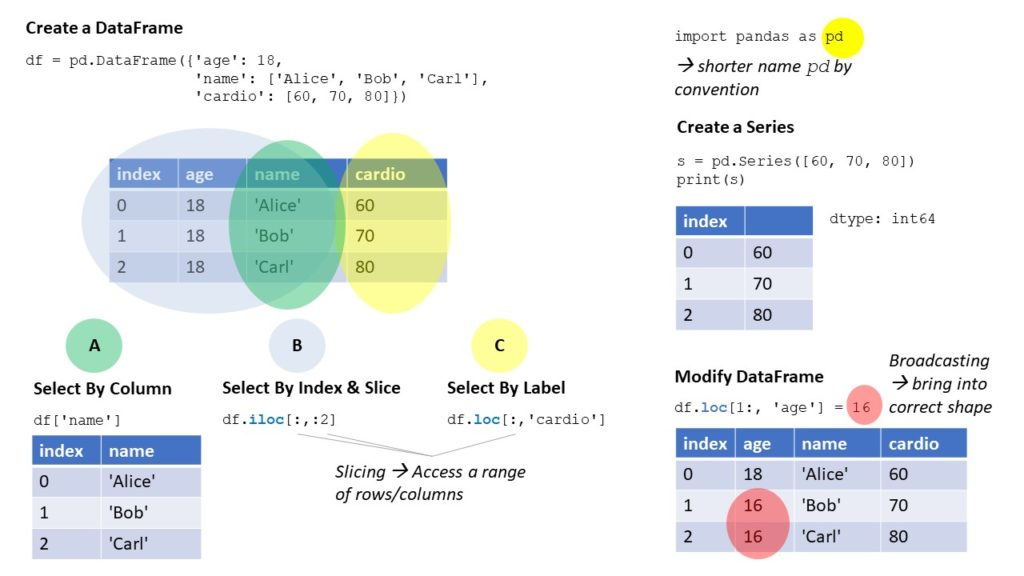
Pandas is a popular Python library for handling and analyzing data. One of the core components in Pandas is the DataFrame object.
A DataFrame can be thought of as a two-dimensional table with columns and rows, which enables the storage and manipulation of data.
Creating a DataFrame is simple, you can start by importing the Pandas library:
import pandas as pd
Now, let’s create a basic DataFrame from a dictionary:
data = {'col1': [1, 2, 3, 4], 'col2': ['A', 'B', 'C', 'D']} df = pd.DataFrame(data)
Here, your DataFrame will look like:
col1 col2 0 1 A 1 2 B 2 3 C 3 4 D
DataFrames have various attributes and functionalities that you can leverage.
- Columns: You can access the column names using
df.columns
which returns an Index object with column labels. - Index: The row labels can be accessed using
df.index
. By default, these are integer-based, but you can set a custom index using theset_index
method. - Shape: To quickly check the number of rows and columns of your DataFrame, use
df.shape
, which returns a tuple (rows, columns).
To select specific parts of your DataFrame, you can use multiple methods:
.loc[]
: Access rows by index label..iloc[]
: Access rows by integer-based index..at[]
: Access a single value using row and column labels..iat[]
: Access a single value using integer-based row and column index.
Additionally, Pandas DataFrame objects offer multiple functionalities like:
- Filtering data based on conditions.
- Performing mathematical operations.
- Merging, concatenating, and joining DataFrames.
- Handling missing or NaN values.
- Grouping data based on specific criteria.
- Sorting data based on column values.
Creating Dataframes
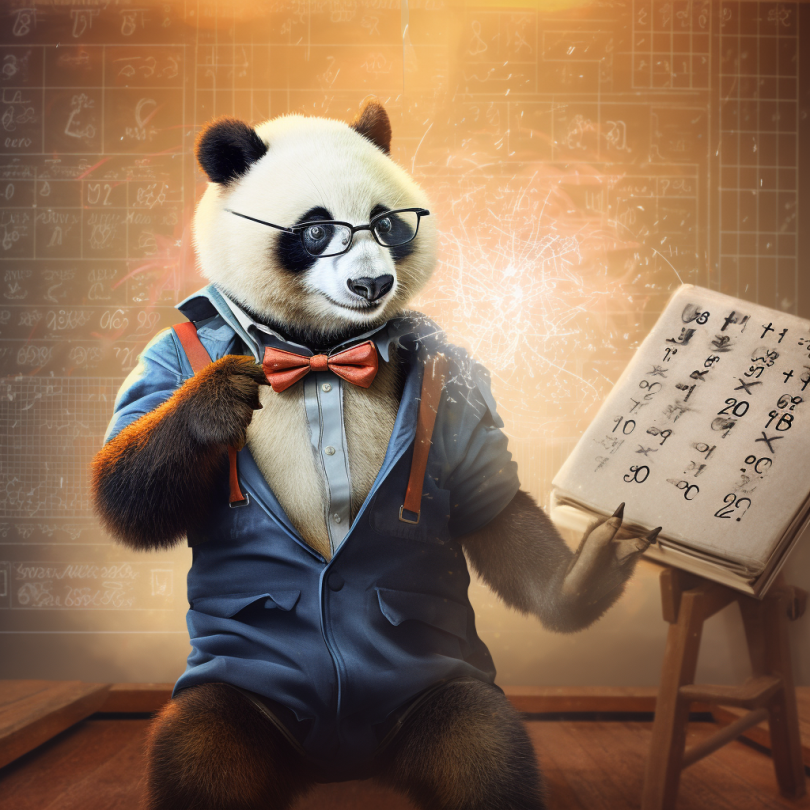
In this section, I’ll cover various ways of creating DataFrames, including from Python dictionaries, Numpy arrays, and CSV files.
From Python Dictionary
You can easily create a DataFrame using a Python dictionary. Keys will become column names, and the values will form the rows. Here’s an example:
import pandas as pd data = { "calories": [420, 380, 390], "duration": [50, 40, 45] } df = pd.DataFrame(data)
This creates a DataFrame with columns calories
and duration
, and rows containing the values specified in the dictionary.
From Numpy Array
Another way to create a DataFrame is by using a Numpy array. First, you need to import the Numpy library and create an array:
import numpy as np array_data = np.array([ [10, 20, 30], [40, 50, 60], [70, 80, 90] ])
Next, you can use the pd.DataFrame()
function to convert the Numpy array to a DataFrame:
df = pd.DataFrame(array_data, columns=["A", "B", "C"])
This creates a DataFrame with columns A
, B
, and C
, and rows containing the values from the Numpy array.
From CSV File
Lastly, you can create a DataFrame from a CSV file. To do this, you can use the pd.read_csv()
function, which reads the CSV file and returns a DataFrame:
csv_df = pd.read_csv("your_file.csv")
Replace "your_file.csv"
with the location and name of your CSV file. This will create a DataFrame containing the data from the CSV file.
Accessing and Manipulating Data

Selecting Rows and Columns
To select specific rows and columns from a DataFrame, you can use the iloc
and loc
methods. The iloc
method is used for integer-based indexing, while loc
is used for label-based indexing.
For example, to select the first row of data using iloc
, you can do:
import pandas as pd # Create a DataFrame data = {'A': [1, 2], 'B': [3, 4]} df = pd.DataFrame(data) # Select the first row using iloc first_row = df.iloc[0]
To select a specific column, you can use the column name as follows:
# Select column 'A' using column name column_a = df['A']
You can also use the loc
method to select rows and columns based on labels:
# Select first row of data using loc with index label first_row_label = df.loc[0]
Slicing
When you need to select a range of rows or columns, slicing comes in handy. Slicing works similarly to Python lists, where you indicate the start and end index separated by a colon. Remember that the end index is exclusive.
For instance, if you want to select the first two rows of a DataFrame, you can slice like this:
# Slice the first two rows first_two_rows = df.iloc[0:2] # Slice the first column using iloc first_column = df.iloc[:, 0:1]
Recommended: Pandas
loc()
and iloc()
– A Simple Guide with Video
Filtering
Filtering the DataFrame can be done using conditions and the query
or eval
method. To filter rows based on specific column values, you can use the query
method:
# Filter rows where column 'A' is greater than 1 filtered_rows = df.query("A > 1")
Alternatively, you can use the eval()
method with conditional statements:
# Filter rows where column 'A' is greater than 1 using eval method condition = df.eval('A > 1') filtered_rows_eval = df[condition]
Modifying Data
You can modify the data in your DataFrame by assigning new values to specific cells, rows, or columns. Directly assigning a value to a cell, using the index and column name:
# Update the value of the cell at index 0 and column 'A' df.loc[0, 'A'] = 5
To modify an entire column or row, simply reassign the new values to the column or row using the column name or index:
# Update the values of column 'A' df['A'] = [6, 7] # Update the values of the first row df.loc[0] = [8, 9]
In summary, you can efficiently access and manipulate the data in your DataFrame using various methods such as iloc
, loc
, query
, eval
, and slicing with indexing.
Indexing and Alignment

Index Objects
In a pandas DataFrame, index objects serve to identify and align your data. Index objects can be created using the Index
constructor and can be assigned to DataFrame columns. They consist of an immutable sequence used for indexing and alignment, making them an essential part of working with pandas.
To create an index object from a Python dict or a list of years, you can use the following code:
import pandas as pd years = [1990, 1991, 1992] index_obj = pd.Index(years) python_dict = {'A': 1, 'B': 2, 'C': 3} index_obj_from_dict = pd.Index(python_dict)
Setting and Resetting Index
To set the index of your DataFrame, use the set_index()
method, which allows you to set one or multiple columns as the index. If you want to revert the index back to a default integer-based index, use the reset_index()
method:
import pandas as pd df = pd.DataFrame({'Year': [2010, 2011, 2012], 'Value': [100, 200, 300]}) # Set the Year column as the index df.set_index('Year', inplace=True) # Reset the index df.reset_index(inplace=True)
Hierarchical Indexing
Hierarchical indexing (also known as multi-level indexing) allows you to create a DataFrame with multiple levels of indexing. You can construct a MultiIndex
using the pd.MultiIndex.from_tuples()
method.
This can help you work with more complex data structures:
import pandas as pd index_tuples = [('A', 'X'), ('A', 'Y'), ('B', 'X'), ('B', 'Y')] multi_index = pd.MultiIndex.from_tuples(index_tuples) # Create a new DataFrame with the MultiIndex columns = ['Value'] data = [[1, 2, 3, 4]] df = pd.DataFrame(data, columns=multi_index)
Alignment and Reindexing
When performing operations on data within a DataFrame, pandas will automatically align the data using the index labels. This means that the order of the data is not important, as pandas will align them based on the index.
To manually align your DataFrame to a new index, you can use the reindex()
method:
import pandas as pd original_index = pd.Index(['A', 'B', 'C']) new_index = pd.Index(['B', 'C', 'D']) # Create a DataFrame with the original index df = pd.DataFrame({'Value': [1, 2, 3]}, index=original_index) # Align the DataFrame to the new index aligned_df = df.reindex(new_index)
Operations on Dataframes
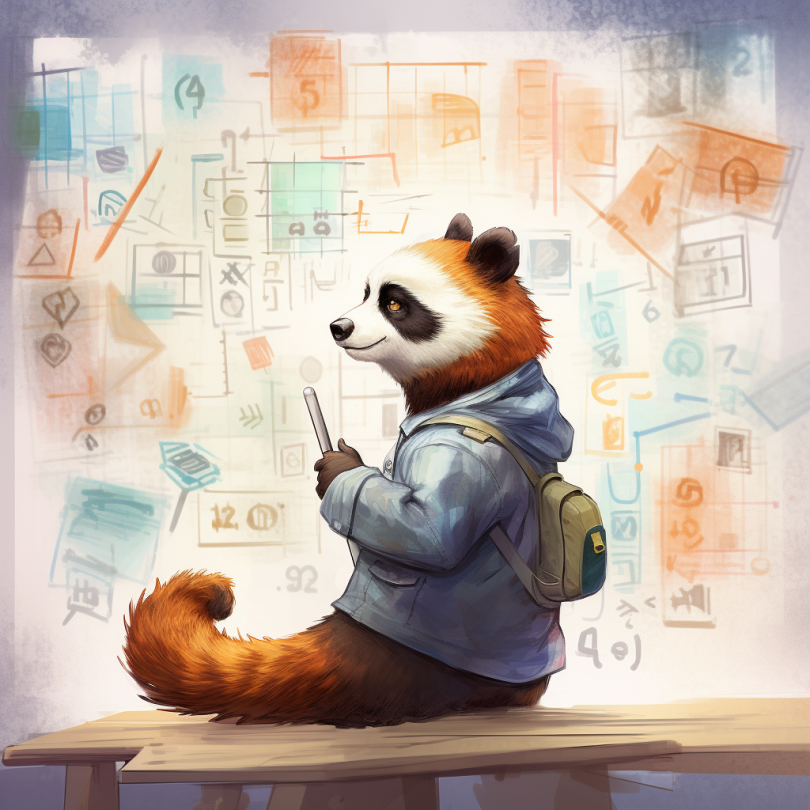
Arithmetic Operations
In pandas, you can easily perform arithmetic operations on DataFrames, such as addition, subtraction, multiplication, and division. Use the pandas methods like add()
and div()
to perform these operations.
For example:
import pandas as pd # Create two DataFrames df1 = pd.DataFrame({'A': [1, 2], 'B': [3, 4]}) df2 = pd.DataFrame({'A': [5, 6], 'B': [7, 8]}) # Perform addition and division result_add = df1.add(df2) result_div = df1.div(df2)
Comparison Operations
You can compare elements in DataFrames using methods like eq()
for equality and compare()
for a more comprehensive comparison. This is useful for tasks like data manipulation and indexing. For instance:
import pandas as pd # Create two DataFrames df1 = pd.DataFrame({'A': [1, 2], 'B': [3, 4]}) df2 = pd.DataFrame({'A': [1, 2], 'B': [3, 5]}) # Perform equality check result_eq = df1.eq(df2) # Perform comprehensive comparison result_compare = df1.compare(df2)
Aggregation Methods
Pandas provides several aggregation methods like sum()
and corr()
to help you summarize and analyze the data in your DataFrame. These methods are especially helpful when dealing with NaN values.
For example:
import pandas as pd import numpy as np # Create a DataFrame with NaN values df = pd.DataFrame({'A': [1, 2, np.nan], 'B': [3, 4, np.nan]}) # Calculate the sum and correlation of each column result_sum = df.sum() result_corr = df.corr()
String Operations
Pandas also supports a wide range of string operations on DataFrame columns, such as uppercasing and counting the occurrences of a substring. To apply string operations, use the .str
accessor followed by the desired operation.
Here’s an example using the upper()
and count(sub)
string methods:
import pandas as pd # Create a DataFrame with string values df = pd.DataFrame({'A': ['apple', 'banana'], 'B': ['cherry', 'date']}) # Convert all strings to uppercase and count the occurrences of 'a' result_upper = df['A'].str.upper() result_count = df['A'].str.count('a')
Handling Missing Data
When working with Pandas DataFrames, it’s common to encounter missing data. This section will cover different techniques to handle missing data in your DataFrames, including detecting null values, dropping null values, and filling or interpolating null values.
Detecting Null Values
To identify missing data in your DataFrame, you can use the isnull()
and notnull()
functions. These functions return boolean masks indicating the presence of null values in your data.
To check if any value is missing, you can use the any()
function, while all()
can be used to verify if all values are missing in a specific column or row.
import pandas as pd # Create a DataFrame with missing values data = {'A': [1, 2, None], 'B': [4, None, 6]} df = pd.DataFrame(data) null_mask = df.isnull() print(null_mask) # Check if any value is missing in each column print(null_mask.any()) # Check if all values are missing in each row print(null_mask.all(axis=1))
Dropping Null Values
If you need to remove rows or columns with missing data, the dropna()
function can help. By default, dropna()
removes any row with at least one null value. You can change the axis parameter to drop columns instead. Additionally, you can use the how
parameter to change the criteria for dropping data.
For example, how='all'
will only remove rows or columns where all values are missing.
# Drop rows with missing values df_clean_rows = df.dropna() # Drop columns with missing values df_clean_columns = df.dropna(axis=1) # Drop rows where all values are missing df_clean_all = df.dropna(how='all')
Filling and Interpolating Null Values
Instead of dropping null values, you can fill them with meaningful data using the fillna()
function. The fillna()
function allows you to replace missing data with a specific value, a method such as forward fill or backward fill, or even fill with the mean or median of the column.
# Fill missing values with zeros df_fill_zeros = df.fillna(0) # Forward fill missing values df_fill_forward = df.fillna(method='ffill') # Fill missing values with column mean df_fill_mean = df.fillna(df.mean())
For a more advanced approach, you can use the interpolate()
function to estimate missing values using interpolation methods, such as linear or polynomial.
# Linear interpolation df_interpolated_linear = df.interpolate() # Polynomial interpolation df_interpolated_polynomial = df.interpolate(method='polynomial', order=2)
Combining Dataframes
In this section, you will learn about three different ways to combine pandas Dataframes: Concatenation, Merging, and Joining.
Concatenation
Concatenation is a simple method of stacking Dataframes either vertically or horizontally. You can use the concatenate
function to achieve this.
import pandas as pd df1 = pd.DataFrame({'A': ['A0', 'A1'], 'B': ['B0', 'B1']}) df2 = pd.DataFrame({'A': ['A2', 'A3'], 'B': ['B2', 'B3']}) result = pd.concat([df1, df2], axis=0, ignore_index=True)
In this example, the Dataframes df1
and df2
have been stacked vertically, and the ignore_index
parameter has been used to reindex the resulting dataframe.
Merging
Merging allows you to combine data based on common columns between your Dataframes. The merge
function allows for various types of merges, such as inner, outer, left, and right.
left = pd.DataFrame({'key': ['K0', 'K1'], 'A': ['A0', 'A1'], 'B': ['B0', 'B1']}) right = pd.DataFrame({'key': ['K0', 'K1'], 'C': ['C0', 'C1'], 'D': ['D0', 'D1']}) result = pd.merge(left, right, on='key')
In this example, the Dataframes left
and right
are merged based on the common column “key.”
Joining
Joining is another method to combine Dataframes based on index. The join
method allows for similar merging options as the merge
function.
left = pd.DataFrame({'A': ['A0', 'A1'], 'B': ['B0', 'B1']}, index=['K0', 'K1']) right = pd.DataFrame({'C': ['C0', 'C1'], 'D': ['D0', 'D1']}, index=['K0', 'K2']) result = left.join(right, how='outer')
In this example, the Dataframes left
and right
are joined based on index, and the how
parameter is set to ‘outer’ for an outer join.
Recommended video and blog article:
How to Merge Pandas DataFrames by Date and Time

Reshaping DataFrames
Reshaping DataFrames is a common task when working with pandas. There are several operations available to help you manipulate the structure of your data, making it more suitable for analysis. In this section, we will discuss pivoting, melting, stacking, and unstacking.
Pivoting
Pivoting is a technique that allows you to transform a DataFrame by reshaping it based on the values of specific columns. In this process, you can specify an index, columns, and values to create a new DataFrame. To perform a pivot, you can use the pivot()
function.
For example:
pivot_df = df.pivot(index='population', columns='instance', values='point')
Here, population
is used as the index, instance
determines the columns, and the values in the point
column will be the new DataFrame’s data. This transformation is helpful when you want to restructure your data for improved readability or further analysis.
Melting
Melting is the opposite of pivoting, where you “melt” or “gather” columns into rows. The melt()
function helps you achieve this. With melting, you can specify a list of columns that would serve as id_vars
(identifier variables) and value_vars
(value variables).
Here’s an example:
melted_df = df.melt(id_vars='population', value_vars=['instance', 'point'])
The melt()
function combines the instance
and point
columns into a single column, while keeping the population
column as the identifier.
Stacking
Stacking is another operation that allows you to convert a DataFrame from a wide to a long format by stacking columns into a multi-level index. You can use the stack()
method to perform stacking.
For instance:
stacked_df = df.stack()
This will create a new DataFrame with a multi-level index where the additional level is formed by stacking the columns of the original DataFrame. This is useful when you want to analyze your data in a more hierarchical manner.
Unstacking
Unstacking is the inverse operation of stacking. It helps you convert a long-format DataFrame with a multi-level index back into a wide format. The unstack()
method allows you to do this. For example:
unstacked_df = stacked_df.unstack()
This will create a new DataFrame where the multi-level index is converted back into columns. Unstacking is useful when you want to revert to the original structure of the data or prepare it for visualization.
Advanced Features
In this section, we’ll explore some advanced features available in Pandas DataFrame, such as Time Series Analysis, Groupby and Aggregate, and Custom Functions with Apply.
Time Series Analysis
Time Series Analysis is a crucial component for handling data with a time component. With Pandas, you can easily work with time-stamped data using pd.to_datetime()
. First, ensure that the index of your DataFrame is set to the date column.
For example:
df['date'] = pd.to_datetime(df['date']) df.set_index('date', inplace=True)
Now that your DataFrame has a DateTimeIndex, you can access various Datetime properties like year
, month
, day
, etc., by using df.index.[property]
. Some operations you can perform include:
- Resampling: You can resample your data using the
resample()
method. For instance, to resample the data to a monthly frequency, executedf.resample('M').mean()
. - Rolling Window: Calculate rolling statistics like rolling mean or rolling standard deviation using the
rolling()
method. For example, to compute a 7-day rolling mean, utilizedf.rolling(7).mean()
.
You can watch our Finxter explainer video here:

Groupby and Aggregate
The groupby()
method helps you achieve powerful data aggregations and transformations. It involves splitting data into groups based on certain criteria, applying a function to each group, and combining the results.
Here’s how to use this method:
- Split: Group your data using the
groupby()
function by specifying the column(s) to group by. For example,grouped_df = df.groupby('type')
. - Apply: Apply an aggregation function on the grouped data, like
mean()
,count()
,sum()
, etc. For instance,grouped_df_mean = grouped_df.mean()
. - Combine: The result is a new DataFrame with the groupby column as the index and the aggregated data.
You can also use the agg()
function to apply multiple aggregate functions at once, or even apply custom functions.
For example:
grouped_df = df.groupby('type').agg({'price': ['mean', 'count'], 'distance': lambda x: list(x)})
Custom Functions with Apply
You can apply custom functions to DataFrame columns or rows with apply()
. This method allows you to apply any function that you define or that comes from an external library like NumPy.

For instance, to apply a custom function to a specific column:
def custom_function(value): # Your custom logic here return processed_value df['new_column'] = df['column'].apply(custom_function)
It’s also possible to apply functions element-wise with applymap()
. This method enables you to process every element in your DataFrame while keeping its structure:
def custom_function_elementwise(value): # Your custom logic here return processed_value df = df.applymap(custom_function_elementwise)
By leveraging these advanced features, you can effectively work with complex data using Pandas DataFrames, while handling operations on Time Series data, group-based aggregations, and custom functions.
Remember to consider features such as dtype
, data types
, loc
, ge
, name
, diff
, count
, strings
, and r
while working with your data, as these can significantly impact your analysis’ efficiency and accuracy.
Optimization and Performance
In this section, we’ll discuss some effective strategies that can help you improve the efficiency of your code.
Data Types Conversion
Use the appropriate data types for your DataFrame columns. Converting objects to more specific data types can reduce memory usage and improve processing speed.
Use the astype()
method to change the data type of a specific column:
df['column_name'] = df['column_name'].astype('new_data_type')
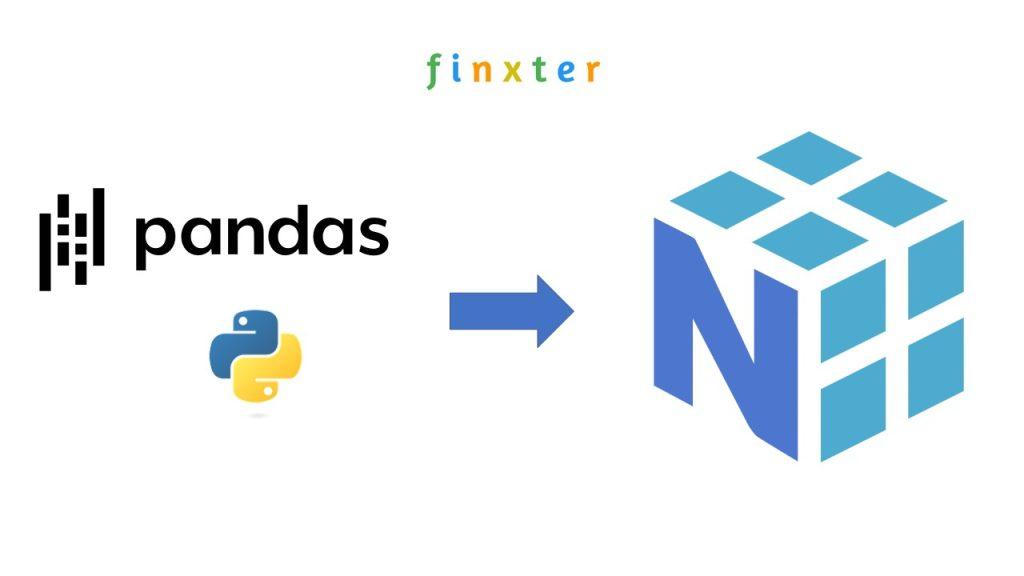
You might also want to consider converting pandas Series to numpy arrays using the to_numpy()
function for intensive numerical computations. This can make certain operations faster:
numpy_array = df['column_name'].to_numpy()
Memory Optimization
Another way to enhance performance is by optimizing memory usage. Check the memory consumption of your DataFrame using the info()
method:
df.info(memory_usage='deep')
For large datasets, consider using the read_csv()
function’s low_memory
,dtype
, usecols
, and nrows
options to minimize memory consumption while importing data:
df = pd.read_csv('file.csv', low_memory=False, dtype={'column1': 'float32', 'column2': 'category'}, usecols=['column1', 'column2'], nrows=100000)
Parallel Processing
Leveraging parallel processing can significantly decrease the time required for computations on large DataFrames. Make use of pandas’ apply()
function in combination with multiprocessing
for parallel execution of functions across multiple cores:
import pandas as pd from multiprocessing import cpu_count, Pool def parallel_apply(df, func): n_cores = cpu_count() data_split = np.array_split(df, n_cores) pool = Pool(n_cores) result = pd.concat(pool.map(func, data_split)) pool.close() pool.join() return result df['new_column'] = parallel_apply(df['column_name'], some_function)
Dask
Dask is a parallel computing library for Python that can significantly speed up pandas’ operations on large DataFrames. Dask’s DataFrame
object is similar to pandas’ DataFrame
, but it partitions the data across multiple cores or even clusters for parallel processing. To use Dask, simply replace your pandas import with Dask:
import dask.dataframe as dd
Then, you can read and process large datasets as you would with pandas:
ddf = dd.read_csv('large_file.csv')
By implementing these optimization techniques, you’ll be able to efficiently work with large DataFrames, ensuring optimal performance and minimizing processing time.
Frequently Asked Questions
How to create a DataFrame from a list?
To create a DataFrame from a list, you can use the pandas.DataFrame()
function. First, import the pandas library:
import pandas as pd
Then, create a list of data:
data = [['A', 10], ['B', 20], ['C', 30]]
Finally, create the DataFrame using the pd.DataFrame()
function:
df = pd.DataFrame(data, columns=['Letter', 'Number']) print(df)
This will create a DataFrame with columns ‘Letter’ and ‘Number’ and the values from the list.
Recommended: How to Create a DataFrame From Lists?
What are some examples of using DataFrames?
DataFrames are versatile data structures that allow for easy manipulation and analysis of data. Some common operations include selecting a specific column or row, filtering data, or applying functions to the data.
For example, to select the ‘Letter’ column:
letters = df['Letter'] print(letters)
Or to filter data based on a specific condition:
filtered_data = df[df['Number'] > 10] print(filtered_data)
How to append data to a DataFrame?
To append data to a DataFrame, first create a new DataFrame with the same columns, then use the append()
method:
new_data = pd.DataFrame([['D', 40]], columns=['Letter', 'Number']) df = df.append(new_data, ignore_index=True) print(df)
This will append the new_data
DataFrame to the original DataFrame.
How to create a DataFrame from a dictionary?
To create a DataFrame from a dictionary, use the pd.DataFrame()
function with the dictionary as the input:
data = {'Letter': ['A', 'B', 'C'], 'Number': [10, 20, 30]} df = pd.DataFrame(data) print(df)
This will create a DataFrame with the keys as column names and the values as the data.
Recommended: 7 Best Ways to Convert Dict to CSV in Python
How to add a row to a DataFrame?
To add a row to a DataFrame, you can use the loc[]
indexer:
df.loc[len(df.index)] = ['E', 50] print(df)
This will add a new row with the values ‘E’ and 50 to the DataFrame.
How to save a DataFrame as CSV file?
To save a DataFrame as a CSV file, use the to_csv()
method:
df.to_csv('data.csv', index=False)
This will save the DataFrame to a CSV file named ‘data.csv’ in your current working directory. The index=False
parameter tells pandas not to include the index column in the CSV file.
Recommended: 10 Minutes to Pandas (in 5 Minutes)
Be on the Right Side of Change